Future Computing and Informatics Journal
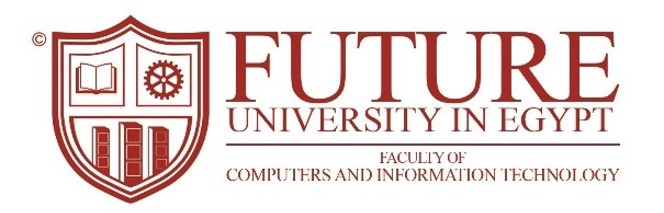
Abstract
Breast cancer is one of the dreadful diseases that affect women globally. The occurrences of breast masses in the breast region are the main cause for women to develop a breast cancer. Early detection of breast mass will increase the survival rate of women and hence developing an automated system for detection of the breast masses will support radiologists for accurate diagnosis. In the pre-processing step, the images are pre-processed using Gaussian filtering. An automated detection method of breast masses is proposed using an optimized region growing technique where the initial seed points and thresholds are optimally generated using a swarm optimization technique called Dragon Fly Optimization (DFO). The texture features are extracted using GLCM and GLRLM techniques from the segmented images and fed into a Feed Forward Neural Network (FFNN) classifier trained using back propagation algorithm which classifies the images as benign and malignant. The performance of the proposed detection technique is evaluated using the images obtained from DDSM database. The results achieved by the proposed pixel-based technique are compared to other region growing methods using ROC analysis. The sensitivity of the proposed system reached up to 98.1% and specificity achieved is 97.8% in which 300 images are used for training and testing purposes.
Recommended Citation
Punitha, S.; Amuthan, A.; and Joseph, K. Suresh
(2018)
"Benign and malignant breast cancer segmentation using optimized region growing technique,"
Future Computing and Informatics Journal: Vol. 3:
Iss.
2, Article 19.
Available at:
https://digitalcommons.aaru.edu.jo/fcij/vol3/iss2/19