Future Computing and Informatics Journal
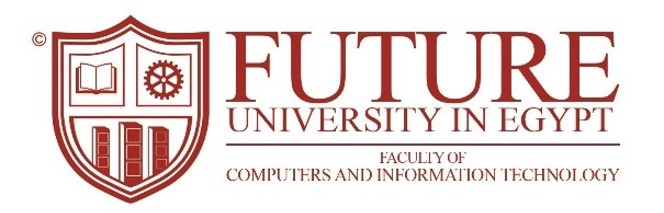
Abstract
Spam emails are not necessary, though they are harmful as they include viruses and spyware, so there is an emerging need for detecting spam emails. Several methods for detecting spam emails were suggested based on the methods of machine learning, which were submitted to reduce non relevant emails and get results of high precision for spam email classification. In this work, a new predictive method is submitted based on antlion optimization (ALO) and boosting termed as ALO-Boosting for solving spam emails problem. ALO is a computational model imitates the preying technicality of antlions to ants in the life cycle. Where ALO was utilized to modify the actual place of the population in the separate seeking area, thus obtaining the optimum feature subset for the better classification submit based on boosting classifier. Boosting classifier is a classification algorithm that points to a group of algorithms which modifies soft learners into powerful learners. The proposed procedure is compared against support vector machine (SVM), k-nearest neighbours algorithm (KNN), and bootstrap aggregating (Bagging) on spam email datasets in a set of implementation measures. The experimental outcomes show the ability of the proposed method to successfully detect optimum features with the smallest value of selected features and a high precision of measures for spam email classification based on boosting classifier.
Recommended Citation
Naem, Amany A.; Ghali, Neveen I. Prof.; and Saleh, Afaf A.
(2018)
"Antlion optimization and boosting classifier for spam email detection,"
Future Computing and Informatics Journal: Vol. 3:
Iss.
2, Article 27.
Available at:
https://digitalcommons.aaru.edu.jo/fcij/vol3/iss2/27