Future Computing and Informatics Journal
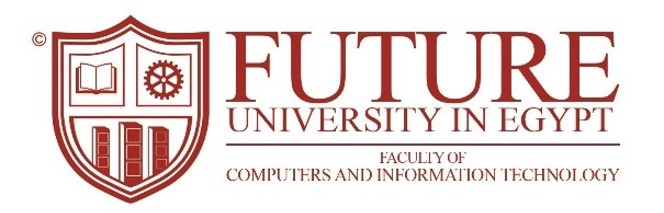
DOI
http://doi.org/10.54623/fue.fcij.6.1.4
Abstract
Forecasting future values of time-series data is a critical task in many disciplines including financial planning and decision-making. Researchers and practitioners in statistics apply traditional statistical methods (such as ARMA, ARIMA, ES, and GARCH) for a long time with varying accuracies. Deep learning provides more sophisticated and non-linear approximation that supersede traditional statistical methods in most cases. Deep learning methods require minimal features engineering compared to other methods; it adopts an end-to-end learning methodology. In addition, it can handle a huge amount of data and variables. Financial time series forecasting poses a challenge due to its high volatility and non-stationarity nature. This work presents a hybrid deep learning model based on recurrent neural network and Autoencoders techniques to forecast commodity materials' global prices. Results show better accuracy compared to traditional regression methods for short-term forecast horizons (1,2,3 and 7 days).
Recommended Citation
Elberawi, Ahmed Saied and Belal, Mohamed Prof.
(2021)
"A Deep Learning Approach for Forecasting Global Commodities Prices,"
Future Computing and Informatics Journal: Vol. 6:
Iss.
1, Article 4.
DOI: http://doi.org/10.54623/fue.fcij.6.1.4
Available at:
https://digitalcommons.aaru.edu.jo/fcij/vol6/iss1/4