Future Computing and Informatics Journal
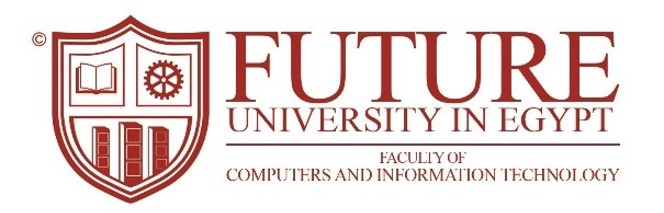
DOI
http://doi.org/10.54623/fue.fcij.6.2.1
Abstract
A feature is a single measurable criterion to an observation of a process. While knowledge discovery techniques successfully contribute in many fields, however, the extensive required data processing could hinder the performance of these techniques. One of the main issues in processing data is the dimensionality of the data. Therefore, focusing on reducing the data dimensionality through eliminating the insignificant attributes could be considered one of the successful steps for raising the applied techniques’ performance. On the other hand, focusing on the applied field, ovarian cancer patients continuously suffer from the extensive analysis requirements for detecting the disease as well as monitoring the treatment progress. Therefore, identifying the most significant required analysis could be a positive step to reduce the emotional and financial suffering. This research aims to reduce the data dimensionality of the ovarian cancer disease and highlight the most significant analysis using the collaboration of clustering techniques and statistical techniques. The research succeeded to identify twelve significant analysis out of forty-four with a total of fourteen significant attributes for ovarian cancer data.
Recommended Citation
Attia, Mohamed; Farghaly, Maha; Hamada, Mohamed; and Idrees, Amira M. AMI
(2021)
"A Statistical-Mining Techniques’ Collaboration for Minimizing Dimensionality in Ovarian Cancer Data,"
Future Computing and Informatics Journal: Vol. 6:
Iss.
2, Article 1.
DOI: http://doi.org/10.54623/fue.fcij.6.2.1
Available at:
https://digitalcommons.aaru.edu.jo/fcij/vol6/iss2/1
Included in
Biomedical Commons, Computer and Systems Architecture Commons, Data Storage Systems Commons