Future Computing and Informatics Journal
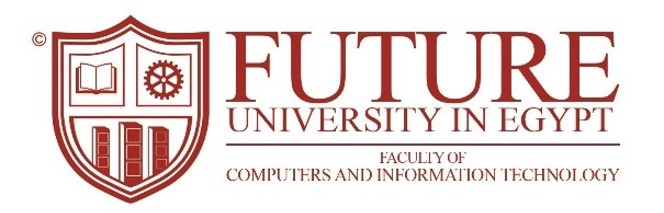
DOI
http://Doi.org/10.54623/fue.fcij.7.1.1
Abstract
Phishing attacks have become one of the most attacks facing internet users, especially after the COVID-19 pandemic, as most organizations have transferred part or most of their work and communication to become online using well-known tools, like email, Zoom, WebEx, etc. Therefore, cyber phishing attacks have become progressively recent, directly and frankly reflecting the designated website, allowing the attacker to observe everything while the victim is exploring Webpages. Hence, utilizing Artificial Intelligence (AI) techniques has become a necessary approach that could be used to detect such attacks automatically. In this paper, we introduce an empirical analysis for automatic phishing detection using several well-known machine learning classification algorithms compared with an ensemble learning model for detecting phishing sites based on the uniform resource locator (URL) using two preprocessed datasets. In this empirical study, we concluded that the ensemble model grants accuracy 97.49% for dataset 1 and 98.69% for dataset 2, which gives higher accuracy than using a single machine learning classification algorithm such as Naive Bayes (NB), Decision Trees (DTs), Random Forest (RF), K-Nearest Neighbors (KNN), Linear Discriminant Analysis (LDA) and Quadratic Discriminant Analysis (QDA). We also compared the proposed ensemble model with one of the most recent similar models
Recommended Citation
Othman, Mahmoud and Hassan, Hesham
(2022)
"An Empirical Study Towards an Automatic Phishing Attack Detection Using Ensemble Stacking Model,"
Future Computing and Informatics Journal: Vol. 7:
Iss.
1, Article 1.
DOI: http://Doi.org/10.54623/fue.fcij.7.1.1
Available at:
https://digitalcommons.aaru.edu.jo/fcij/vol7/iss1/1