Future Computing and Informatics Journal
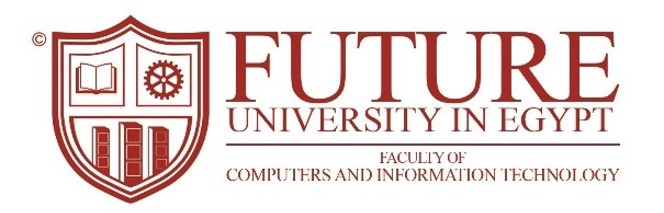
DOI
https://doi.org/10.54623/fue.fcij.7.2.1
Abstract
Feature Selection (FS) is an efficient technique use to get rid of irrelevant, redundant and noisy attributes in high dimensional datasets while increasing the efficacy of machine learning classification. The CSA is a modest and efficient metaheuristic algorithm which has been used to overcome several FS issues. The flight length (fl) parameter in CSA governs crows' search ability. In CSA, fl is set to a fixed value. As a result, the CSA is plagued by the problem of being hoodwinked in local minimum. This article suggests a remedy to this issue by bringing five new concepts of time dependent fl in CSA for feature selection methods including linearly decreasing flight length, sigmoid decreasing flight length, chaotic decreasing flight length, simulated annealing decreasing flight length, and logarithm decreasing flight length. The proposed approaches' performance is assessed using 13 standard UCI datasets. The simulation result portrays that the suggested feature selection approaches overtake the original CSA, with the chaotic-CSA approach beating the original CSA and the other four proposed approaches for the FS task.
Recommended Citation
Abdullahi, Mohammed; Adamu, Abdulhameed; and Hassan, Ibrahim Hayatu
(2022)
"Crow search algorithm with time varying flight length strategies for feature selection,"
Future Computing and Informatics Journal: Vol. 7:
Iss.
2, Article 1.
DOI: https://doi.org/10.54623/fue.fcij.7.2.1
Available at:
https://digitalcommons.aaru.edu.jo/fcij/vol7/iss2/1