International Journal of Thin Film Science and Technology
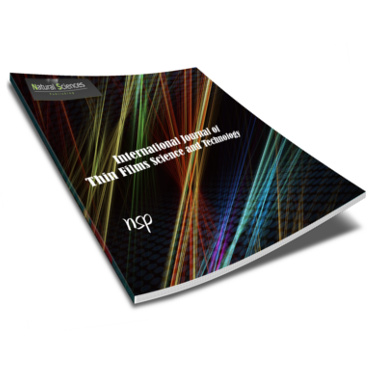
Abstract
Any human being has unique biological traits biological characteristics which can be studied using Biometrics which encompasses an individuals characteristics like DNA, face, finger prints, voice, signatures etc. Human faces as an element of authentication are being increasingly used where biometrics add value in terms of quantifying an individual’s natural data. Facial authentications validate personal identities based on facial images with 1-1 matches. These kinds of authenticating applications have been applied in a variety of areas including banking applications and personal mobile devices. RTFAs (Real Time Face Authentications) based systems are a necessity for ATMs (Automated Teller Machines) in banking for enhanced security. Several machine learning methods have been introduced RTFA based systems. The overall performance of the traditional machine learning methods is lesser due to considering the same image for authentication; noises presented in the image samples. To solve this issues, in this work authentication is performed based on the reconstructed image via deep learning method. The major novelty of the work is to apply a deep learning method for image reconstruction and authentication. None of the existing methods will apply deep learning methods for reconstructing of image with real time authentication. This work proposes a novel supervised DLT (Deep Learning Technique) based on SDAE (Stacked Deep Auto Encoder) for image reconstructions in RTFA based systems. The proposed system consists of five major steps: (1) database collection, (2) Pre-processing, (3) SDAE modelling and image reconstruction, (4). RTFA based system, and (5) Performance evaluation. For the first step, 220 faces are collected in real time from 5 persons (each 44) with image size of 92*92. In the second step, facial images, RGB (Red Green Blue) images are converted to Gray scale which is resized into 32*32-pixel images. In the third step, SDAE model reconstructs the image which is then used by the RTFR system to identify a person. The reconstructed facial image is then compared with previously registered images using threshold based NCCs (Normalized Cross Correlations). The proposed SDAE model is evaluated for reconstructions of the original facial images in terms of PSNRs (Peak Signal To Noise Ratios), MSEs (Mean Square Errors), and RMSEs (Root Mean Square Errors). The proposed SADE classifier gives lesser RMSE results of 0.1000 whereas other methods such as CNN, LSTM and VGG16 gives increased MSE results of 0.3500, 0.30741 and 0.27423.
Recommended Citation
A. Dar, Showkat and Palanivel, S.
(2022)
"Real Time Face Authentication System Using Stacked Deep Auto Encoder for Facial Reconstruction,"
International Journal of Thin Film Science and Technology: Vol. 11
:
Iss.
1
, PP -.
Available at:
https://digitalcommons.aaru.edu.jo/ijtfst/vol11/iss1/9