Journal of Engineering Research
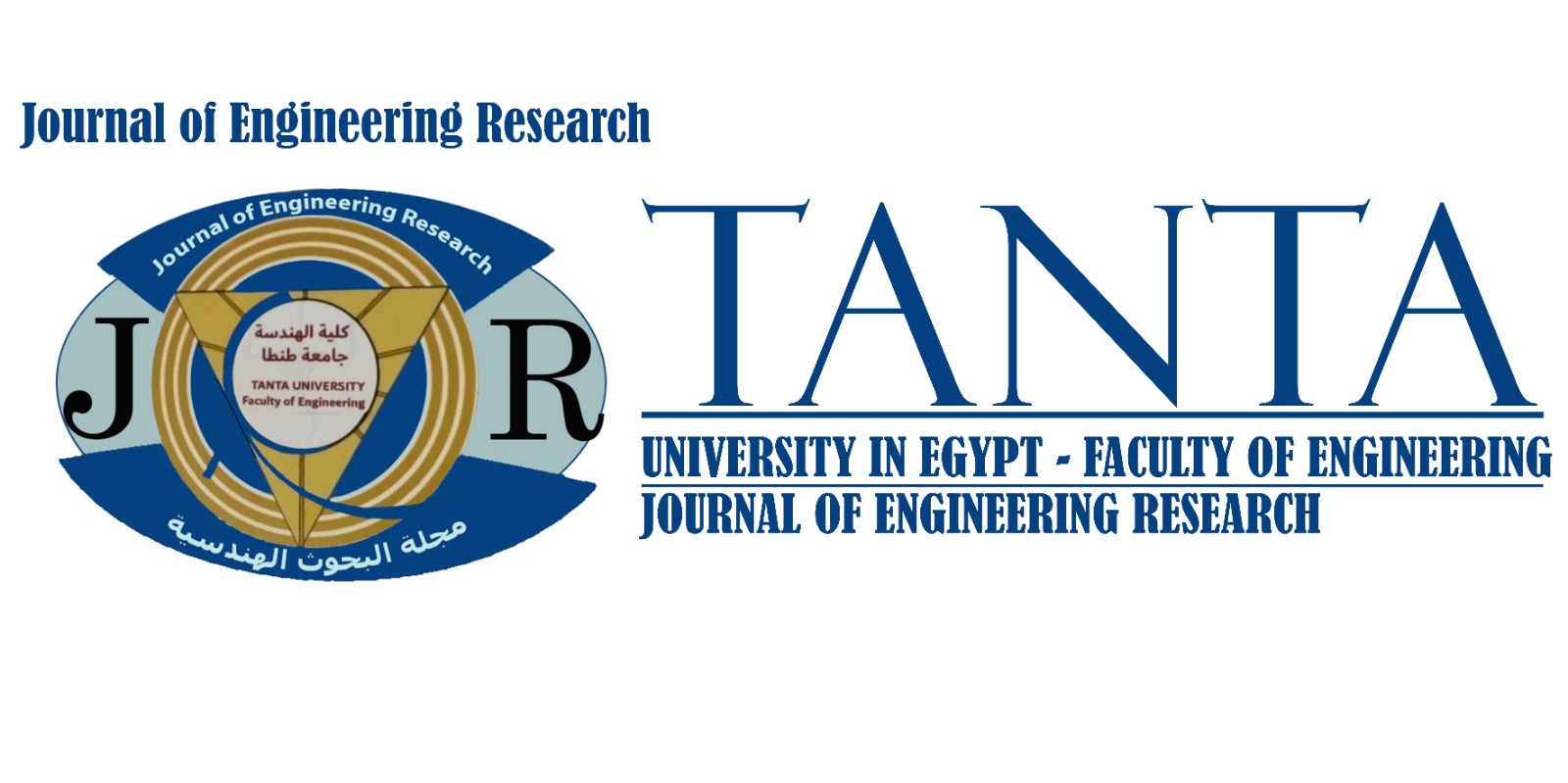
Abstract
Aging significantly affects human health and the overall economy, yet understanding of the underlying molecular mechanisms remains limited. Among all human genes, almost three hundred and five have been linked to human aging. While certain subsets of these genes or specific aging-related genes have been extensively studied. There has been a lack of comprehensive examination encompassing the entire set of aging-related genes. Here, the main objective is to overcome understanding based on an innovative approach that combines the capabilities of deep learning. Particularly using One-Dimensional Deep AutoEncoder (1D-DAE). Followed by the K-means clustering technique as a means of unsupervised learning. The proposed technique offers a novel approach for identifying new candidate proteins with compelling computational evidence of their significant roles in aging. The 1D-DAE model was trained on recognized aging proteins and subsequently employed to effectively compress and represent the complete array of features inherent in all human proteins. This transformation reduced the feature space from 21,000 dimensions to a mere 64 dimensions. Leveraging the distinct characteristics of the aging proteins uncovered during the training phase. Subsequently, we applied the K-means algorithm to partition the human proteins into distinct clusters based on their feature similarities. Using these clusters, we made highly accurate predictions about proteins that may play a significant role in the aging process. Among these proteins, some lack previous annotations related to aging, making the results particularly significant in illuminating potential key proteins in the aging process.
Recommended Citation
Hammad, Sondos M.; Saidahmed, Mohamed Talaat; Sallam, Elsayed A.; and Elbasiony, Reda
(2024)
"Exploring Human Aging Proteins Based on Deep Autoencoders and K-Means Clustering,"
Journal of Engineering Research: Vol. 8:
Iss.
1, Article 3.
Available at:
https://digitalcommons.aaru.edu.jo/erjeng/vol8/iss1/3
Included in
Applied Mathematics Commons, Biological Engineering Commons, Other Computer Engineering Commons