Journal of Engineering Research
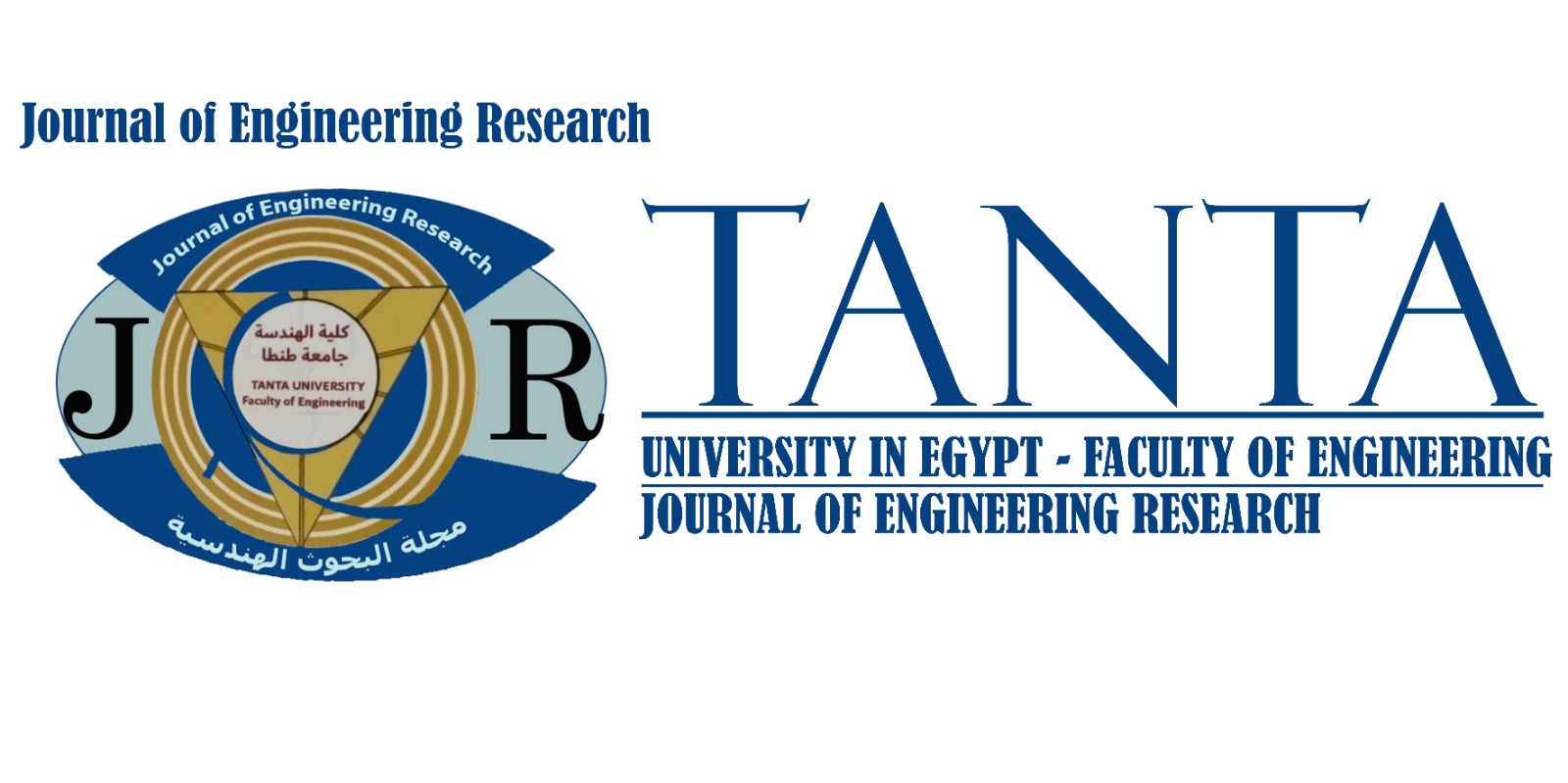
Abstract
In the field of machine learning, the confusion matrix is a specific table adopted to describe and assess the performance of a classification model (e.g. an artificial neural network) for a set of test data whose actual distinguishing features are known. The learning algorithm is thus of the supervised learning category. For an n-class classification problem, the confusion matrix is square with n rows and n columns. The rows represent the class actual samples (instances) which are the inputs to the classifier, while the columns represent the class predicted samples, the classifier outputs. (The converse is also valid, i.e. the two dimensions 'actual' and 'predicted' can be assigned to columns and rows, respectively). Binary as well as multiple-class classifiers can be dealt with. It is worth noting that the term 'matrix' here has nothing to do with the theorems of matrix algebra; it is regarded just as an information-conveying table. The descriptive word ‘confusion’ stems from the fact that the matrix clarifies to what extent the model confuses the classes — mislabels one as another. The essential concept was introduced in 1904 by the British statistician Karl Pearson (1857 — 1936).
Recommended Citation
Fahmy Amin, Mahmoud
(2022)
"Confusion Matrix in Binary Classification Problems: A Step-by-Step Tutorial,"
Journal of Engineering Research: Vol. 6:
Iss.
5, Article 1.
Available at:
https://digitalcommons.aaru.edu.jo/erjeng/vol6/iss5/1