Journal of Engineering Research
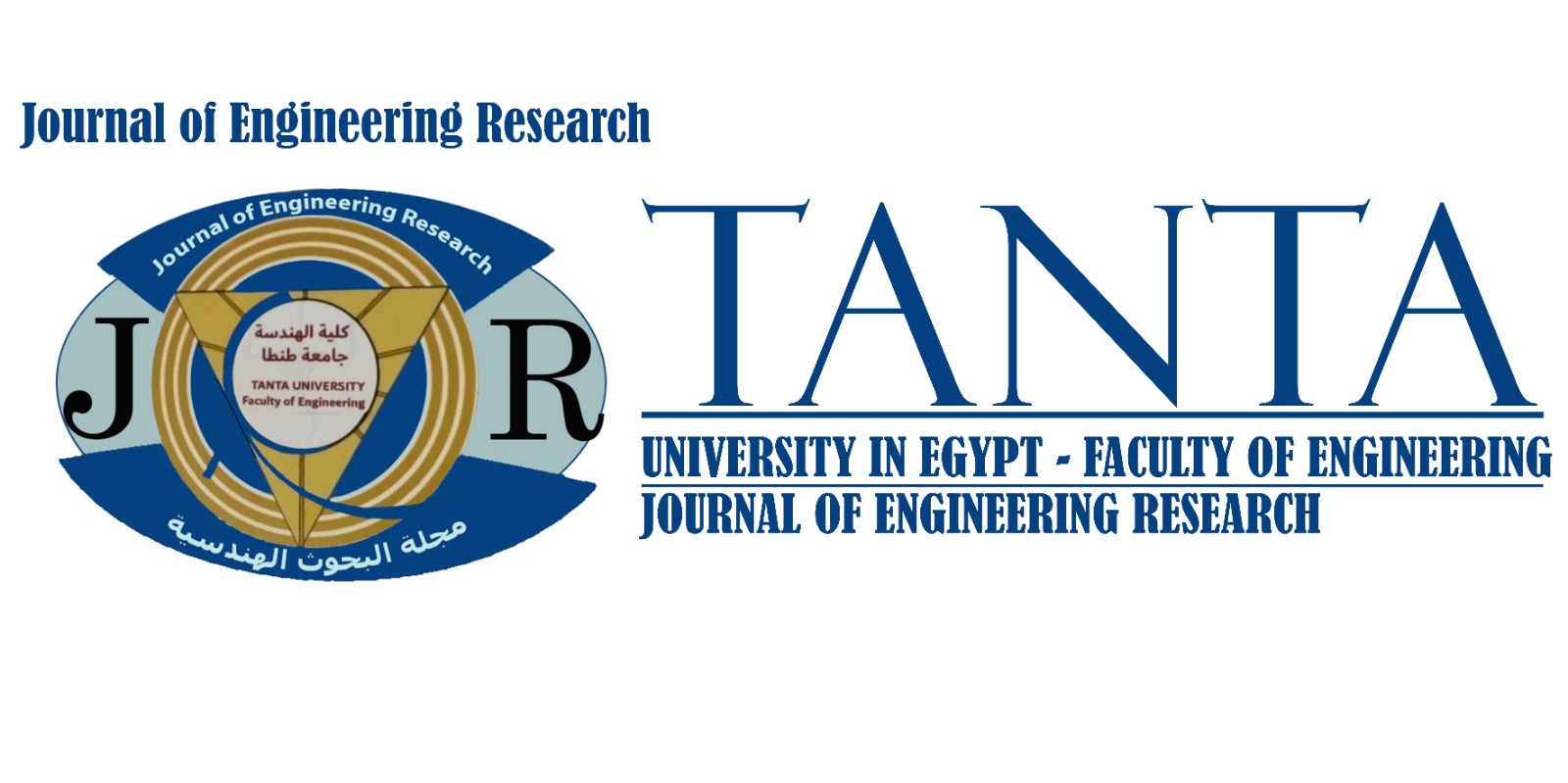
Abstract
The automatic detection of outliers in time series datasets has captured much amount of attention in the data science community. It is not a simple task as the data may have several perspectives, such as sessional, trendy, or a combination of the two. Furthermore, to obtain a reliable and untrustworthy knowledge from the data, the data itself should be understandable. To cope with these challenges, in this paper, we introduce a new framework that can first test the stationarity and seasonality of dataset, then apply a set of Fourier transforms to get the Fourier sample frequencies, which can be used as a support of a decomposer component. The proposed framework, namely TTDD (Test, Transform, Decompose, and Detection), implements the decomposer component that split the dataset into three parts: trend, seasonal, and residual. Finally, the frequency difference detector compares the frequency of the test set to the frequency of the training set determining the periods of discrepancy in the frequency considering them as outlier periods.
Recommended Citation
Khattab, Abdel Hamid
(2023)
"T2D2: A Time Series Tester, Transformer, and Decomposer Framework for Outlier Detection,"
Journal of Engineering Research: Vol. 7:
Iss.
1, Article 18.
Available at:
https://digitalcommons.aaru.edu.jo/erjeng/vol7/iss1/18