Journal of Engineering Research
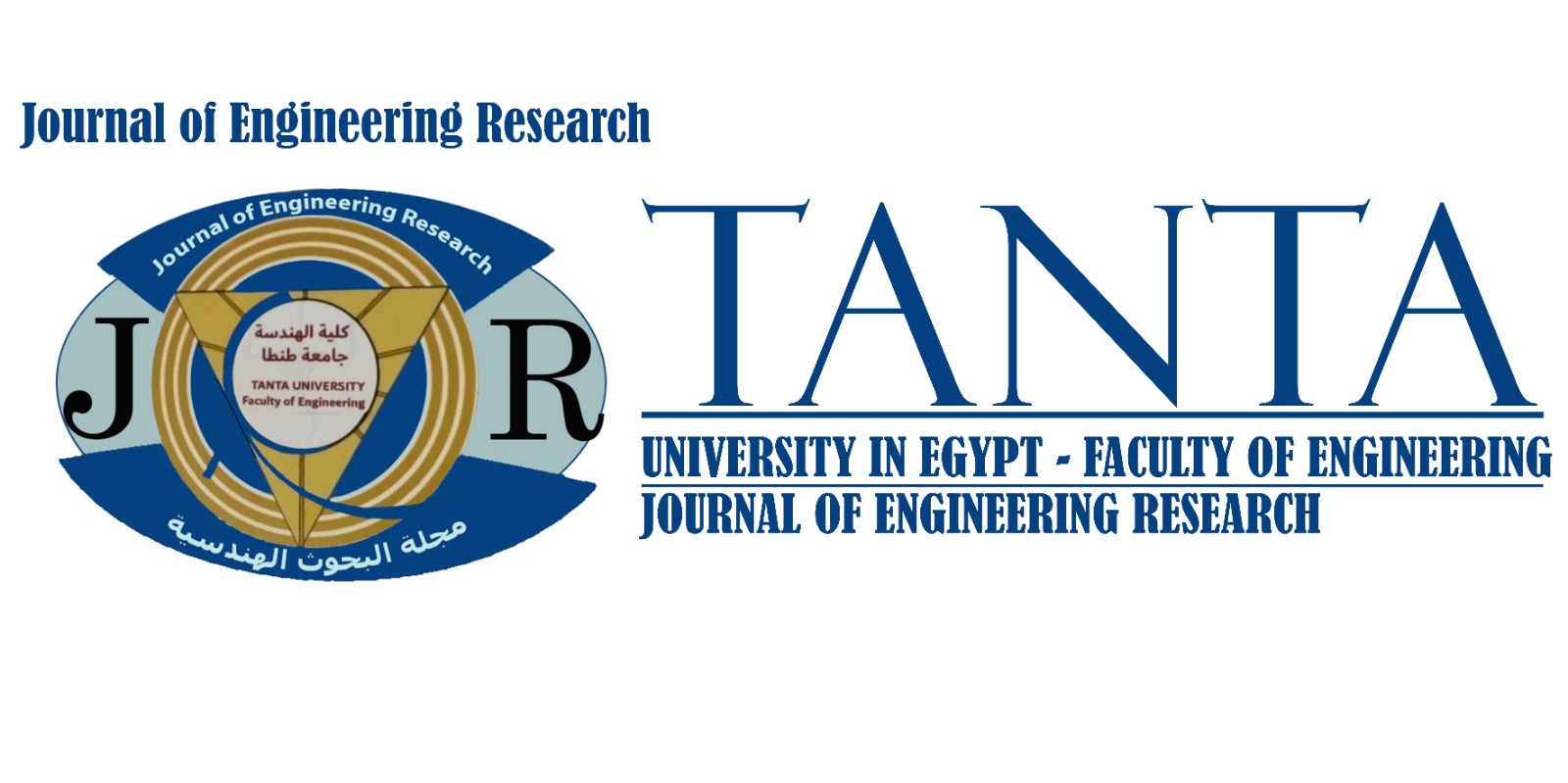
Abstract
Facial expressions are caused by specific movements of the face muscles; they are regarded as a visible manifestation of a person's inner thought process, internal emotional states, and intentions. A smile is a facial expression that often indicates happiness, satisfaction, or agreement. Many applications use smile detection such as automatic image capture, distance learning systems, interactive systems, video conferencing, patient monitoring, and product rating. The smile detection system is divided into two stages: feature extraction and classification. As a result, the accuracy of smile detection is dependent on both phases. In recent years, numerous researchers and scholars have identified various approaches to smile detection, however, their accuracy is still under the desired level. To this end, we propose an effective Convolutional Neural Network (CNN) architecture based on modified LeNet-5 Network (MLeNet-5) for detecting smiles in images. The proposed system generates low-level face identifiers and detect smiles using a strong binary classifier. In our experiments, the proposed MLenet-5 system used the SMILEsmilesD and (GENKI-4 K) databases in which the smile detection rate of the proposed method improves the accuracy by 2% on SMILEsmilesD database and 5% on GENKI-4 K database relative to LeNet-5-based CNN network. In addition, the proposed system decreases the number of parameters compared to LeNet-5-based CNN network and most of the existing models while maintaining the robustness and effectiveness of the results.
Recommended Citation
Maher Obaya, Hend
(2023)
"Deeply Smile Detection Based on Discriminative Features with Modified LeNet-5 Network,"
Journal of Engineering Research: Vol. 7:
Iss.
2, Article 4.
Available at:
https://digitalcommons.aaru.edu.jo/erjeng/vol7/iss2/4