Journal of Engineering Research
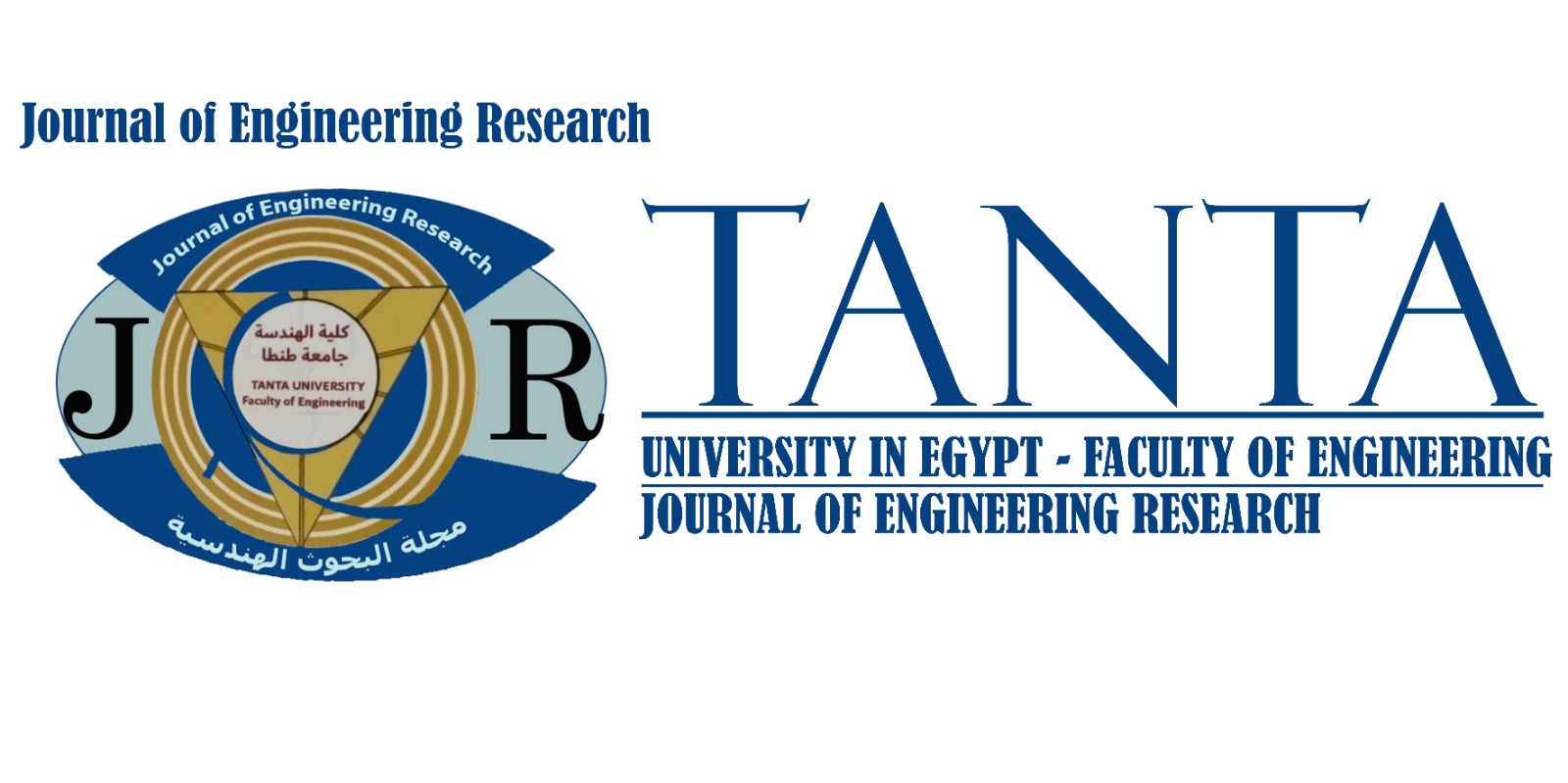
Abstract
Network slicing empowers service providers to deploy diverse network slice architectures within a shared physical infrastructure. This technology enables the provision of differentiated services that cater for specific Quality of Service (QoS) requirements of different use cases which need to be adequately supported in 5G networks. By leveraging Network Slicing, operators can effectively meet these diverse requirements and provide customized services to different tenants in a flexible and efficient manner. However, infrastructure providers face a challenging dilemma of the slice admission control regarding whether to accept or reject slice requests. From one perspective, they strive to optimize the utilization of network resources through accepting a significant number of network slices. From another perspective, the availability of network resources is restricted, and it is crucial to fulfil the QoS requirements specified by the network slices. In this research, an Admission Control (AC) Algorithm founded upon Reinforcement Learning mechanisms, specifically Q-Learning (QL), Double Q-Learning (Double-QL), and a proposed mechanism based on Double QL is obtained to overcome this challenge. This algorithm is applied in order to make informed decisions regarding network slice requests. The simulation results demonstrate that the AC algorithm, leveraging the suggested mechanism, surpasses the Double-QL and QL mechanisms in relation to gained profit with average of 8% and 26%, respectively. In case of the acceptance ratio of slice requests, it achieves average of 13% and 28% higher than Double-QL and QL mechanisms, respectively. Finally, it obtains the maximum resource utilization, surpassing Double-QL and QL by 9% and 20%, respectively.
Recommended Citation
Ibrahim, Mohamed TALAAT FAHIM, Nada Elshennawy, Mai Z.
(2023)
"Slice Admission control based on Reinforcement Learning for 5G Networks,"
Journal of Engineering Research: Vol. 7:
Iss.
3, Article 12.
Available at:
https://digitalcommons.aaru.edu.jo/erjeng/vol7/iss3/12