Journal of Engineering Research
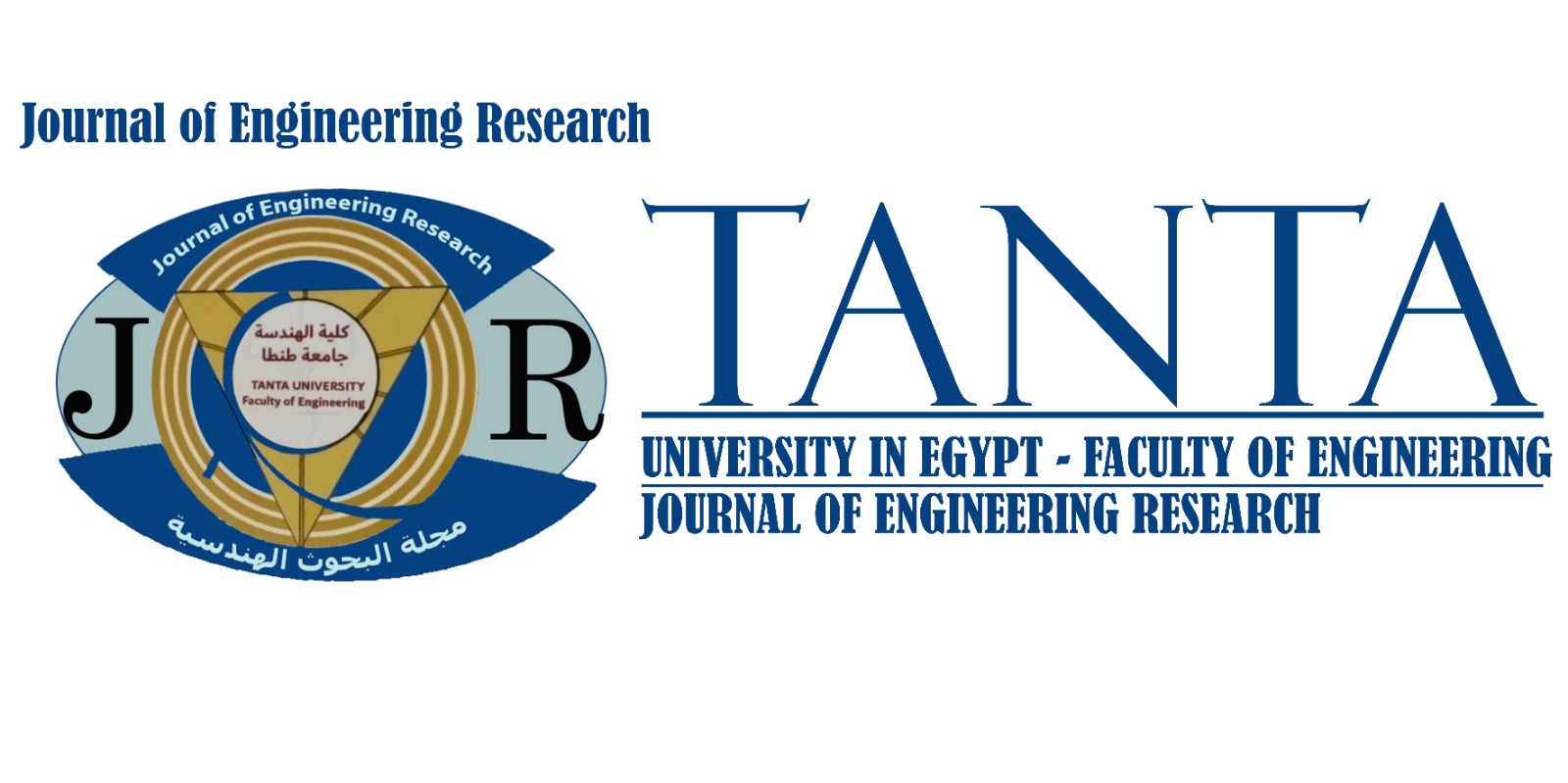
Abstract
Accurate and prompt detection of system faults are crucial to maintain sufficient protection of system equipment, avoid false tripping, and cascaded failures.This paper presents a comprehensive study on the effectiveness of machine learning techniques for electrical fault detection and classification. Specifically, a comparative analysis is conducted between two prominent algorithms: Recurrent Neural Networks (RNNs) and Decision Tree (DT). The study employs a dataset comprising real-world electrical fault scenarios to evaluate the performance of RNNs and DT in identifying and categorizing faults. While DT algorithm showed slightly better accuracy in some cases, the RNN exhibited better generalization capabilities and a lower risk of overfitting. The analysis involves various performance metrics such as accuracy, precision, recall, and confusion matrices to comprehensively assess the algorithms' capabilities. The findings provide valuable insights into the strengths and limitations of each approach in the context of electrical fault management. This paper contributes to the selection of suitable techniques based on specific application requirements, advancing the field of predictive maintenance and fault mitigation in electrical systems. Keywords- Decision Tree, Electrical Faults, Fault Classification, Fault Detection, Machine Learning, Recurrent Neural Networks.
Recommended Citation
Abuanwar, Mohammed Saeed, Hanan Mosalem, Hatem Khater, Sayed
(2023)
"Recurrent Neural Networks RNNs and Decision Tree DT Machine Learning-Based Approaches For Transmission System Faults Diagnosis,"
Journal of Engineering Research: Vol. 7:
Iss.
5, Article 10.
Available at:
https://digitalcommons.aaru.edu.jo/erjeng/vol7/iss5/10