Journal of Engineering Research
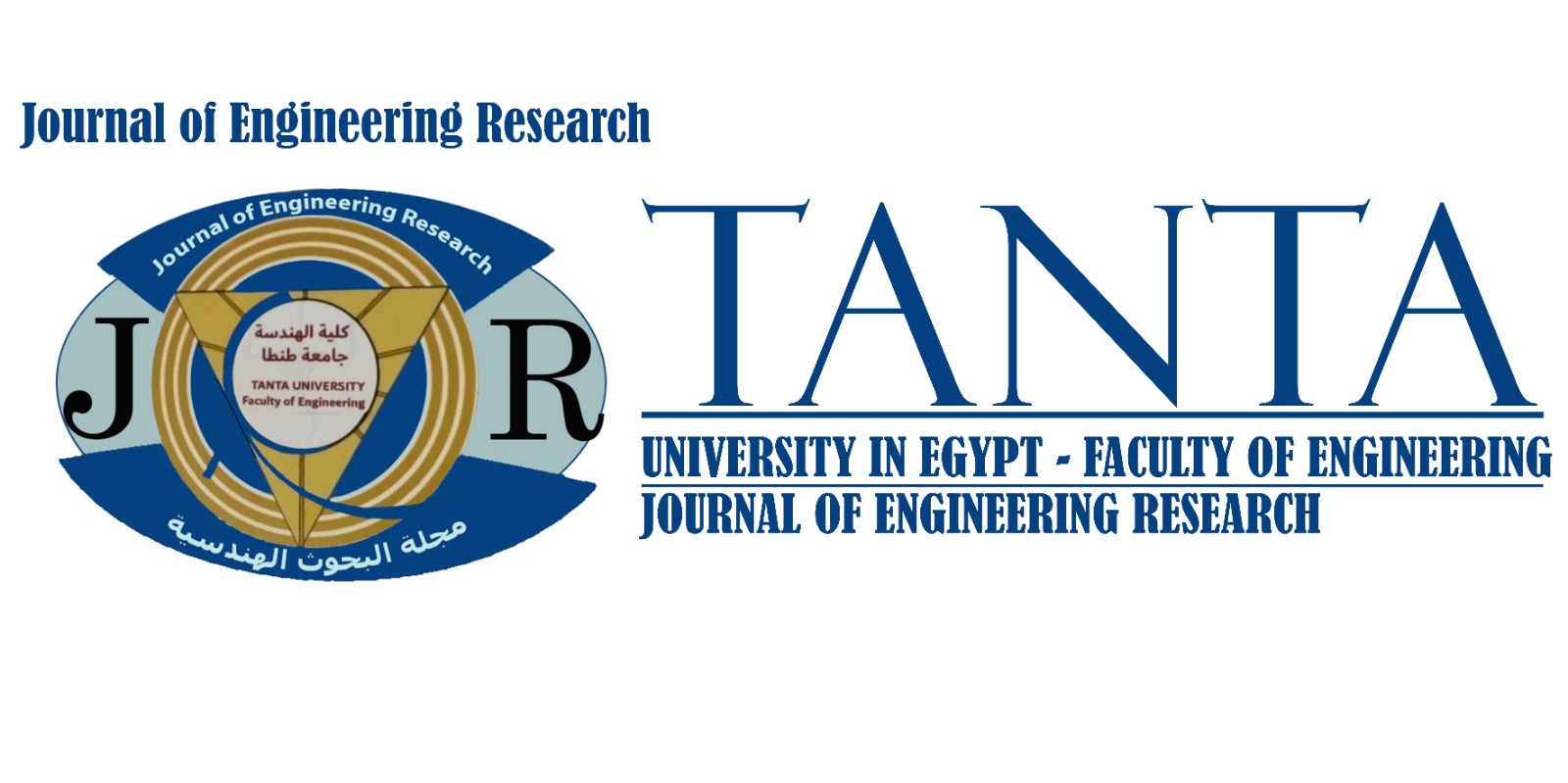
Abstract
Sign Language (SL) recognition plays a crucial role in facilitating communication and inclusivity for the hearing-impaired community. In this paper, we introduce a novel approach that incorporates two distinct activation functions—Exponential Linear Unit (ELU) and HardSwish-ELU—to enhance recognition using the pre-trained YOLOv5 object recognition model. ELU is integrated into the intermediate layers of the YOLOv5 architecture, addressing the vanishing gradient problem and capturing more expressive features. The HardSwish activation function, known for combining the advantages of ReLU and LeakyReLU, is employed for the final classification layer to enhance discriminative power. Throughout the training process, we use the SGD-based optimizer to further boost the model's performance. Our models outperform state-of-the-art models, benefiting from reduced training parameters, overhead, and computational costs. Various experiments were conducted on the ArSL21L dataset, a large-scale Arabic sign language dataset, to assess the proposed fusion of activation functions in terms of accuracy, precision, and recall. The combination of ELU and HardSwish enables better representation learning, allowing the model to capture intricate sign language gestures more accurately. This breakthrough has the potential to advance Arabic sign language recognition and contribute to creating inclusive technologies for the hearing-impaired community. The proposed model's effectiveness was further assessed using the OkkhorNama: BdSL dataset and ASLL (American Sign Language Letters) dataset, demonstrating significant improvements in recognition rates compared to the baseline YOLOv5 model with conventional activation functions. The proposed model achieved maximum levels of accuracy, recall, mAP 0.5, and mAP 0.5:0.95 at 98.2%, 98.5%, 99.1%, and 84.4%, respectively. Finally, the proposed model was tested in a real-time environment under various conditions, demonstrating its reliability and efficacy. This comprehensive research contributes to the advancement of sign language recognition technology, fostering inclusivity and communication for the hearing-impaired community.
Recommended Citation
Attia, Nehal F.; Said ahmed, Mohamed Talaat Faheem; and Alshewimy, Mahmoud A. M.
(2024)
"Improved Deep Learning Model based Real-Time Recognition of Arabic Sign Language,"
Journal of Engineering Research: Vol. 8:
Iss.
1, Article 31.
Available at:
https://digitalcommons.aaru.edu.jo/erjeng/vol8/iss1/31