Journal of Engineering Research
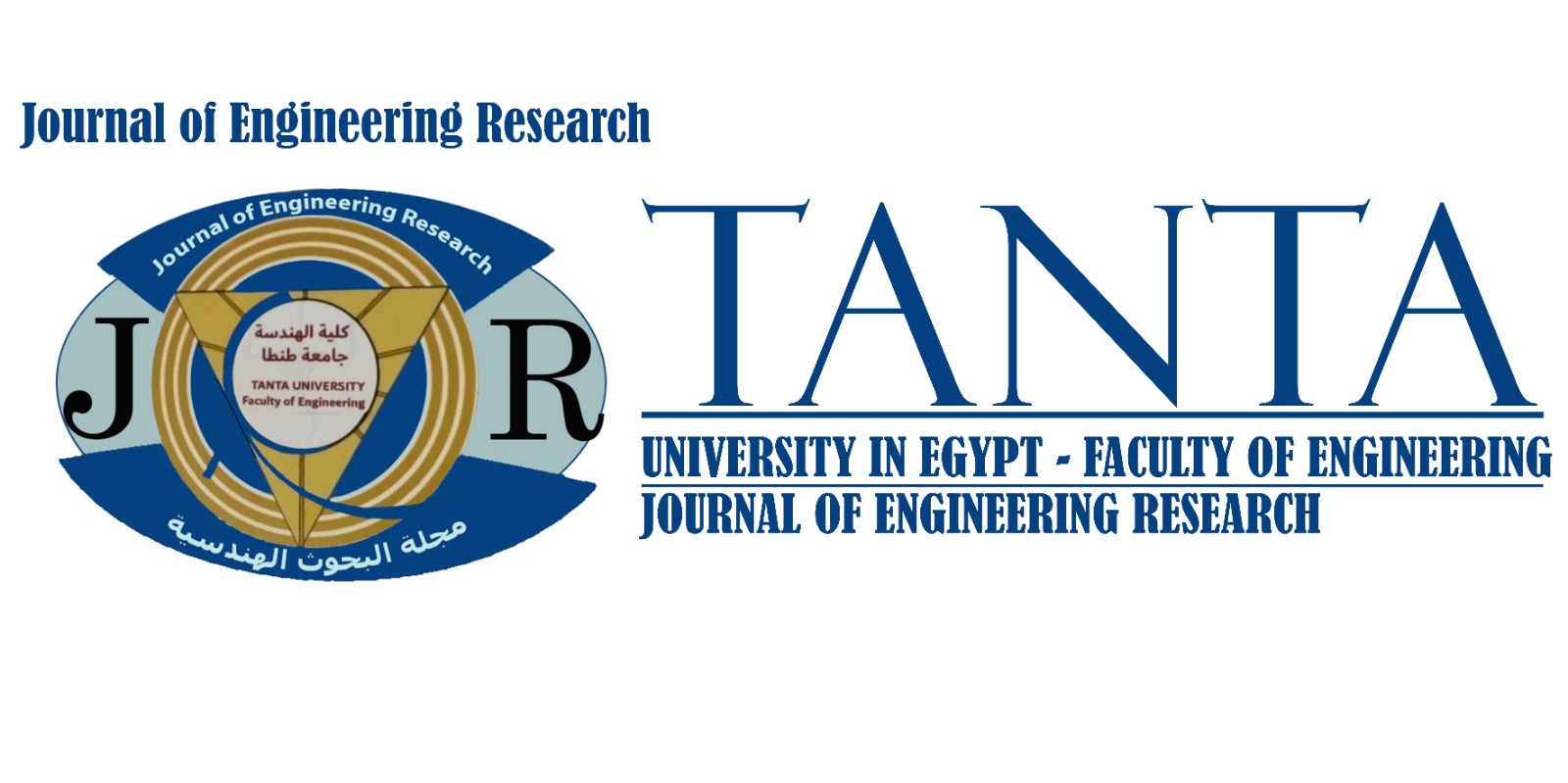
Abstract
The proliferation of fake news has become a complex and challenge problem in recent year, and presenting various unsolved issues within the research domain. Among these challenges, a critical concern is the development of effective models capable of accurately distinguish between fake and real news. While numerous techniques have been proposed for fake news detection, achieving optimal accuracy remains elusive. This paper introduces a novel fake news detection approach employing a two-layered weighted voting classifier. In contrast to conventional methods that assign equal weights to all classifiers, our proposed approach utilizes a selective weighting approach to solve the current issue. The simplicity of equal weight voting classifiers, being easy to implement and resource-efficient, forms the basis of our initial layer. However, rather than uniform weights, a selective weighting technique is employed, assigning higher weights to classifiers demonstrating superior performance on a validation set. The system utilizes five base classifiers in first layer to generate individual predictions. These predictions are combined using a soft voting scheme, where the probabilities of each prediction are weighted at first layer. Stacking is introduced, refining predictions through a final layer soft voting classifier. To boost the performance, the feature selection method via grid search is implemented. Moreover, the system is evaluated the approach on two benchmark datasets and compare it with several state-of-the-art methods. The experimental results show that the proposed method achieves 4.2% and 5% higher accuracy, outperforming other methods that only use a single classifier. This underscores the effectiveness of the two-layered weighted voting classifier in addressing the intricate challenges of fake news detection.
Recommended Citation
Wynne, Hnin Ei and Swe, Khaing Thanda
(2024)
"A Soft Two-Layers Voting Model for Fake News Detection,"
Journal of Engineering Research: Vol. 8:
Iss.
1, Article 4.
Available at:
https://digitalcommons.aaru.edu.jo/erjeng/vol8/iss1/4