Journal of Engineering Research
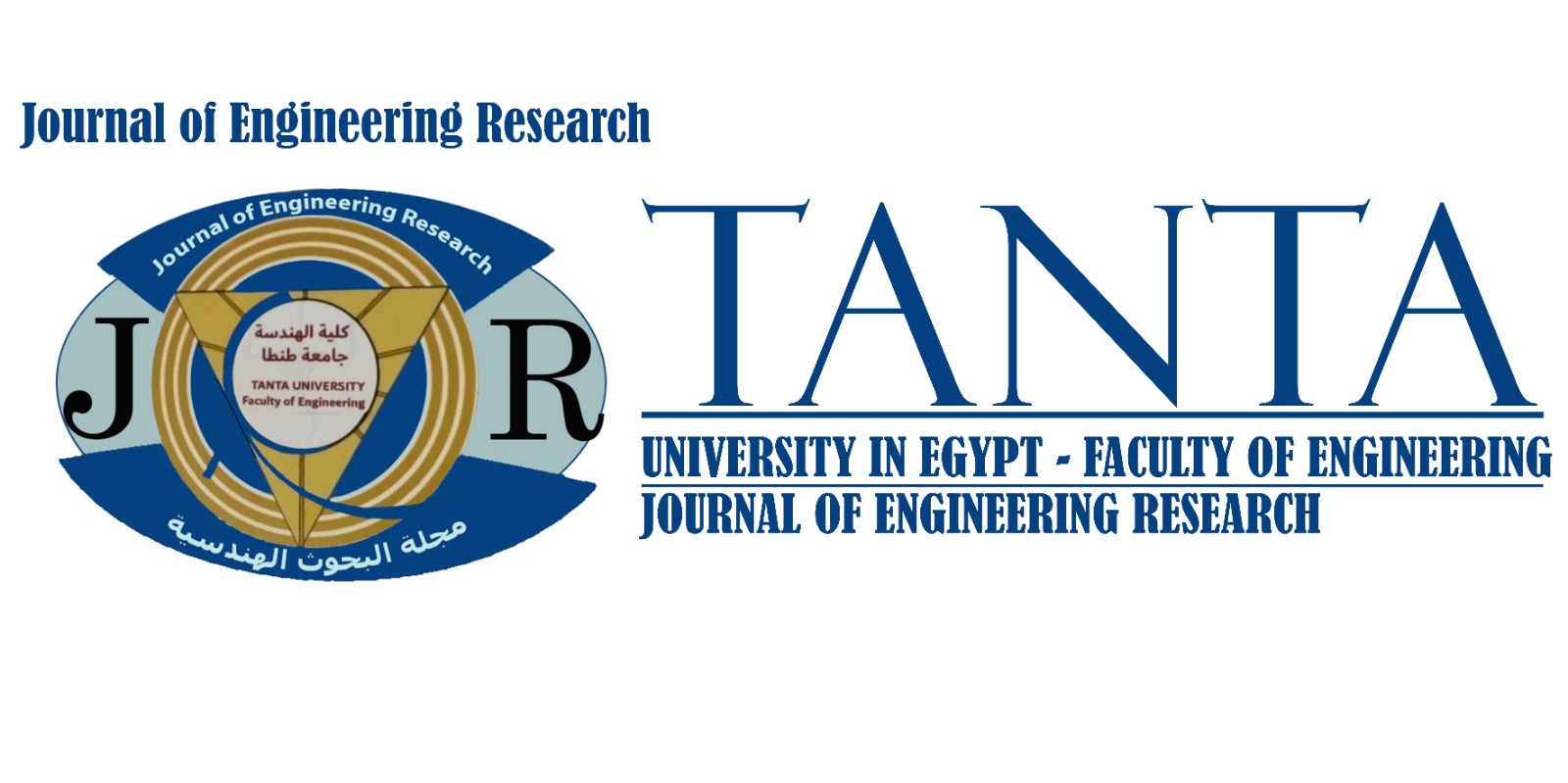
Abstract
Aging is a major risk factor for numerous chronic diseases and declines in physical and cognitive functions, making it a critical focus of research. Identifying biomarkers that signify biological aging is crucial for developing interventions that can potentially delay age-related diseases. Among these biomarkers, human aging proteins are of significant interest. However, laboratory experiments to discover aging proteins are complex, costly, and time-consuming. Often focusing on single proteins at a time. To address this challenge, we used a clustering algorithm to detect potential human aging proteins. This approach aims to aid biologists in their experimental efforts. Deep clustering, particularly simultaneous deep clustering, offers a powerful approach in this context. We employ the Structural Deep Clustering Network (SDCN) in this approach. It concurrently utilizes representation learning and structural learning with deep clustering. The Bio-SDCN model is designed to cluster human proteins into potential aging and non-aging categories by integrating five essential algorithms: K-nearest neighbors (KNN), Graph Convolutional Network (GCN), autoencoder (AE), K-means clustering, and Bi-Self Training Deep Clustering (Bi-STDC). It operates across three stages—Preparation, Learning, and Inference—to effectively analyze and categorize the human proteins. In the Learning stage, the Bio-SDCN model first trains an AE to initialize AE and cluster centers. During the Learning stage, AE and GCN refine protein representations and clustering, with Bi-STDC further enhancing this process. The Inference stage employs the trained model to predict about 1.5% new potential aging proteins in a larger dataset, demonstrating nearly 92% accuracy, an F-score of 83.53%, a precision of 77.96%, and a clustering loss of 0.0124. These results highlight the model's effectiveness in identifying potential aging biomarkers. While these results indicate the model’s potential for identifying aging proteins, they are preliminary and meant to guide further experimental validation. The use of Bio-SDCN model offers a robust framework for advancing research into aging and related biomarkers.
Recommended Citation
Hammad, Sondos M.; Saidahmed, Mohamed Talaat; Sallam, Elsayed A.; and Elbasiony, Reda
(2024)
"Enhancing Human Aging Proteins Detection Based on Bio-Structural Deep Clustering Network,"
Journal of Engineering Research: Vol. 8:
Iss.
4, Article 36.
Available at:
https://digitalcommons.aaru.edu.jo/erjeng/vol8/iss4/36
Included in
Biomedical Informatics Commons, Engineering Commons, Health Information Technology Commons