Journal of Engineering Research
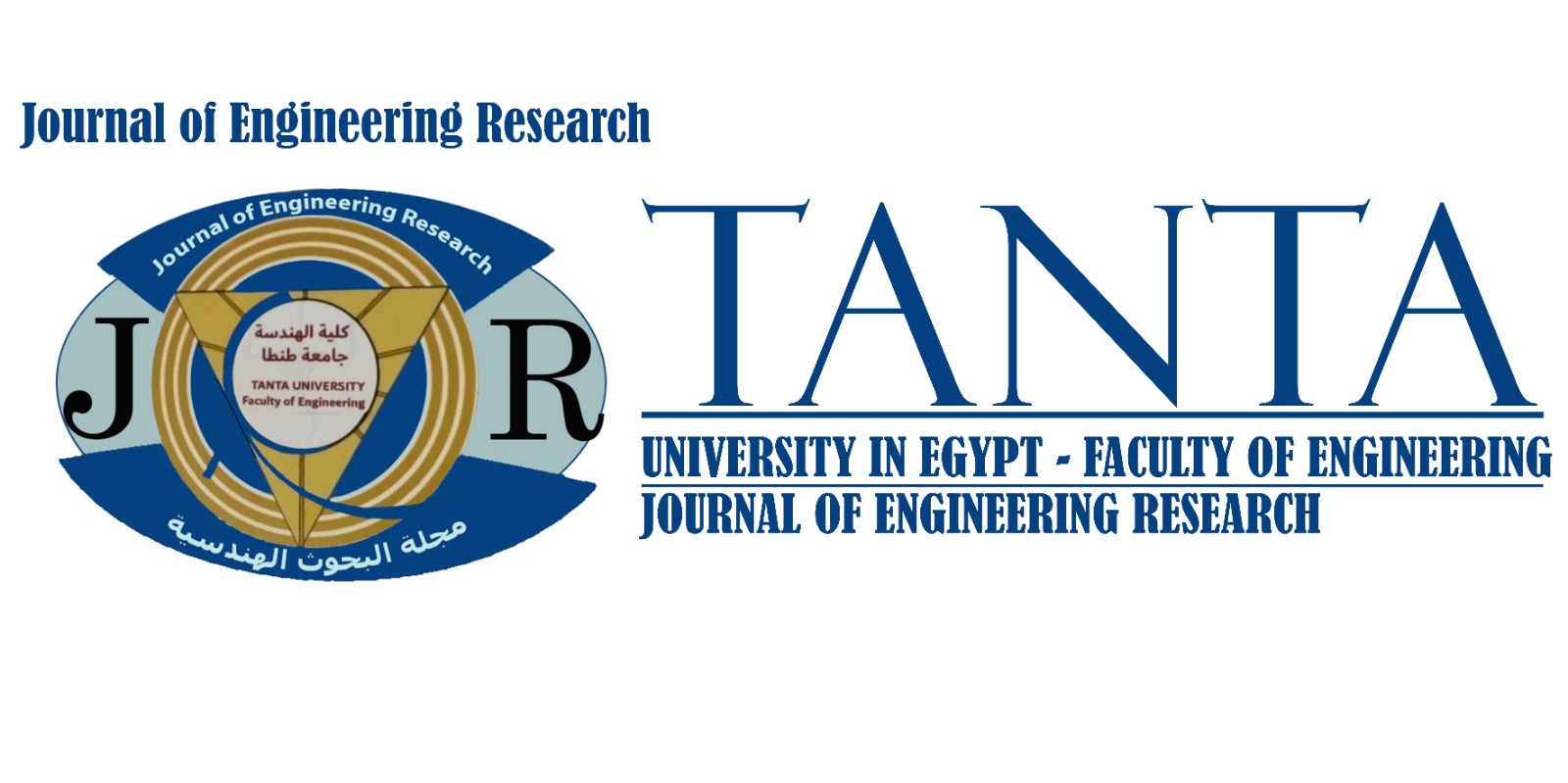
DOI
https://doi.org/10.70259/engJER.2025.911912
Abstract
The detection of plant diseases a vital task for ensuring crop health and optimizing agricultural productivity. Machine learning and computer vision have significantly advanced the automation of plant disease detection. However, traditional methods face several challenges, particularly with data annotation and the limitations of task-specific models, which often fail to generalize across different plant diseases. These methods are hindered by their reliance on models tailored to individual crops and the ongoing need for manual inspections, leading to inefficiencies and restricted scalability. This study proposes a method designed to enable the efficient and accurate identification of a broad range of plant diseases based on residual network-101 integrated with efficient channel attention mechanism. The residual network effectively learns deep features from plant images, while the efficient channel attention mechanism emphasizes relevant features in the network, improving the model’s focus and robustness. To evaluate the effectiveness of our approach, we trained and tested our model using the widely recognized PlantVillage dataset, known for its diverse and comprehensive representation. Our method achieved an accuracy of 99.45%. Additionally, when tested on new, unseen datasets, the model reached an accuracy of 98.45%, demonstrating its robustness and ability to generalize effectively to unfamiliar data.
Recommended Citation
Hagar, Asmaa Aly; Bastwesy, Marwa Reda; Elbasiony, Reda; and Saidahmed, Mohamed Talaat
()
"A Generalized Plant Disease Detection Technique Based On Residual Network With Efficient Channel Attention,"
Journal of Engineering Research: Vol. 9:
Iss.
1, Article 21.
DOI: https://doi.org/10.70259/engJER.2025.911912
Available at:
https://digitalcommons.aaru.edu.jo/erjeng/vol9/iss1/21