Journal of Engineering Research
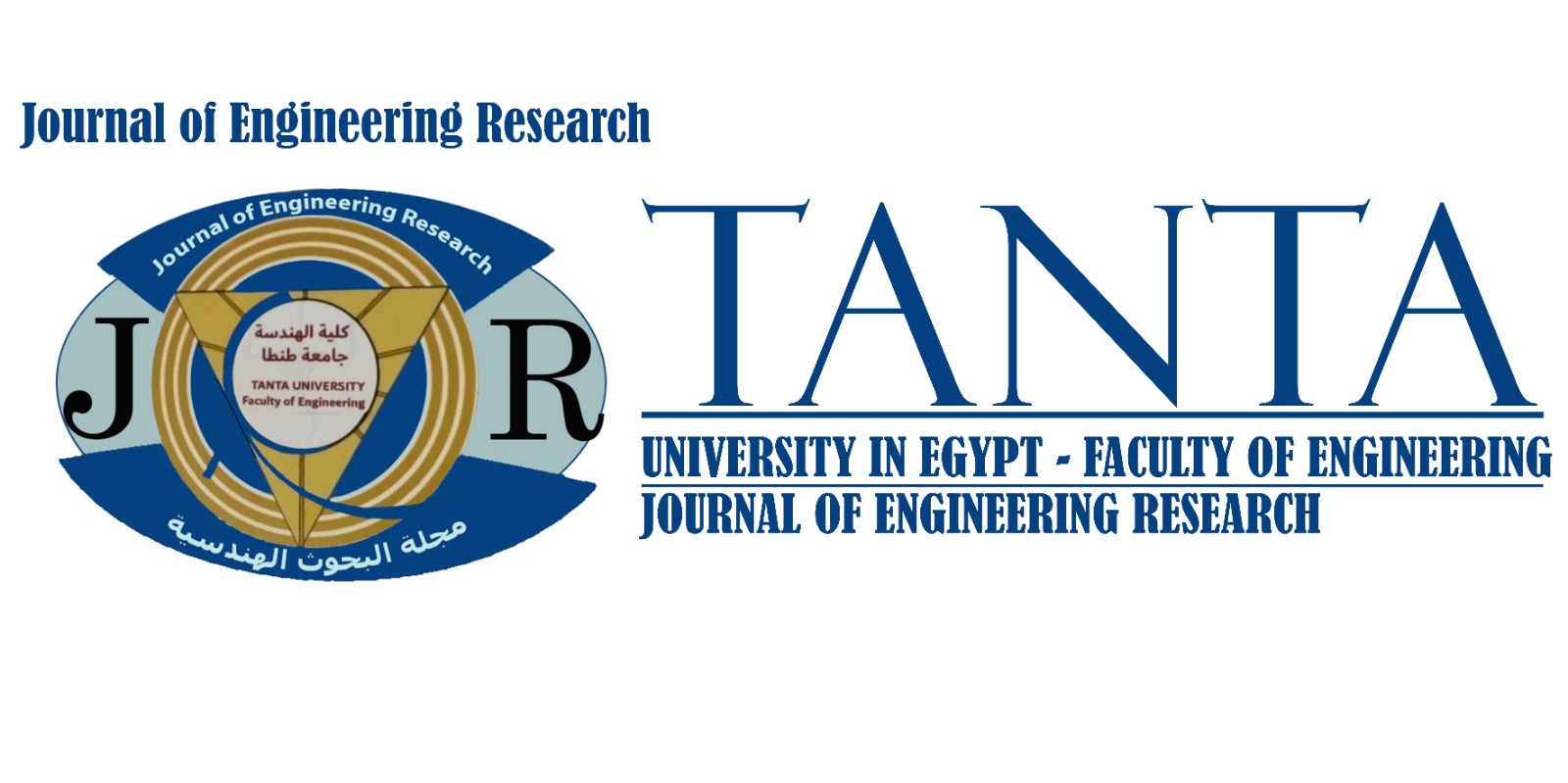
DOI
https://doi.org/10.70259/engJER.2025.911931
Abstract
Early and accurate detection of plant leaf diseases is crucial for safeguarding agricultural productivity and ensuring food security. Traditional methods of plant disease detection, which often rely on manual inspections and specialized models, encounter challenges such as limited scalability, data annotation difficulties, and task-spe-cific constraints. This paper introduces two innovative and general-ized approaches for plant disease detection by combining Residual Networks with channel attention modules. The first approach inte-grates ResNet-101, the Efficient Channel Attention (ECA) mecha-nism, and the Squeeze-and-Excitation Network (SENet), while the second combines ResNetRS-101 with the ECA mechanism. These models leverage the strengths of ResNets, the dynamic channel atten-tion offered by ECA, and the global context modeling provided by SE-Net, forming a robust and generalized solution for identifying plant diseases across various species. The models were evaluated using the PlantVillage dataset, achieving accuracies of 99.37% and 99.5%, re-spectively. Furthermore, when tested on novel and previously unseen datasets, the models demonstrated their resilience and generalization capabilities, attaining accuracies of 99.22% and 98.45%, respectively, proving their ability to effectively handle unfamiliar data.
Recommended Citation
Hagar, Asmaa Aly; Bastwesy, Marwa Reda; Elbasiony, Reda; and Faheem, Mohamed Talaat
()
"Generalized Plant Disease Detection Using Residual Networks with ECA and SENet Integration,"
Journal of Engineering Research: Vol. 9:
Iss.
1, Article 35.
DOI: https://doi.org/10.70259/engJER.2025.911931
Available at:
https://digitalcommons.aaru.edu.jo/erjeng/vol9/iss1/35